Machine failure refers to the unexpected malfunction or breakdown of a mechanical system, often resulting in operational disruptions, downtime, and the need for repairs. It can be caused by various factors, including mechanical wear, electrical issues, or software failures, impacting overall efficiency and productivity.
The Challenges
Potential Failures in Manufacturing Industries include Heat Dissipation Failure, Random Failures, Tool Wear Failure, Power Failure, Overstrain Failure, or No Failure scenarios.
Complexity in predicting various failure modes accurately. Heat dissipation failures may require monitoring temperature fluctuations. Random failures are inherently unpredictable. Tool wear failures may involve continuous monitoring of equipment conditions.
Power failures may need real time power consumption analysis. Overstrain failures may be linked to load monitoring. The absence of failure may depend on establishing a baseline of normal operations.
Involved training algorithms on historical data related to machine performance and failure instances. The model learns patterns and correlations to make predictions about potential failures in real time.
Enhances equipment reliability, reduces downtime, and optimizes maintenance efforts. Ultimately improves overall operational efficiency and user experience.
What did PredictEasy do..
Types of failures considered include problems with heat, random malfunctions, worn out tools, power issues, too much strain, or instances with no failure.
The model analyzes features like temperature, tool conditions, and power use from the dataset to understand machine operations.
Statistical tests, such as Pearson Correlation Coefficient and Spearman Rank Correlation, help understand connections between different features.
The Chi-Square Test examines connections between categories, revealing how specific features relate to different types of failures and ANOVA test identifies significant differences between groups, providing insights into variations in different manufacturing situations.
Analyses offer a clear picture of the manufacturing process, aiding in smart decision making and proactive maintenance planning.
The insights derived help find ways to reduce the chances of failures in manufacturing. A PredictEasy model was developed and a real time interface with a What-If Simulator, allowing users to input data and get the probability of failure.
The model includes AI-powered actionable insights for informed decision making in response to dynamic situation.
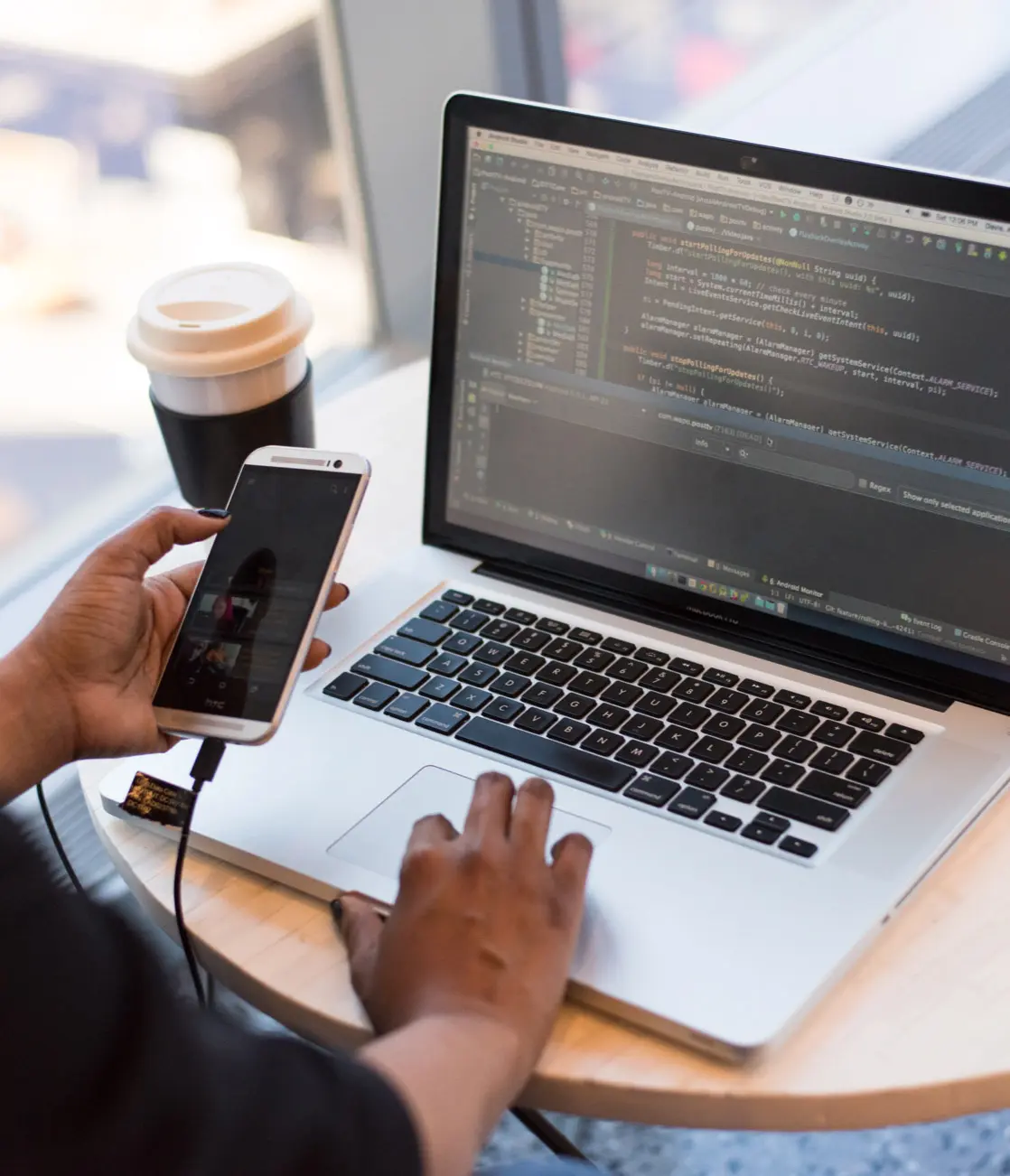
The Results
Torque demonstrates a statistically significant positive correlation with a particular type of failure, suggesting a moderate association. Rotational speed, Tool wear, Machine type, Process temperature, and Air temperature do not exhibit a strong correlation with the type of failure. Air temperature and Process temperature is a likely similarity in distribution, implying no substantial difference between the two attributes.
The predictive model demonstrates exceptional performance in predicting Failure Type, with high accuracy, precision, recall, and F1 score. Torque is a crucial factor, exhibiting a significant impact on predicting Failure Type. Rotational speed and tool wear are important features contributing to the model's accuracy.
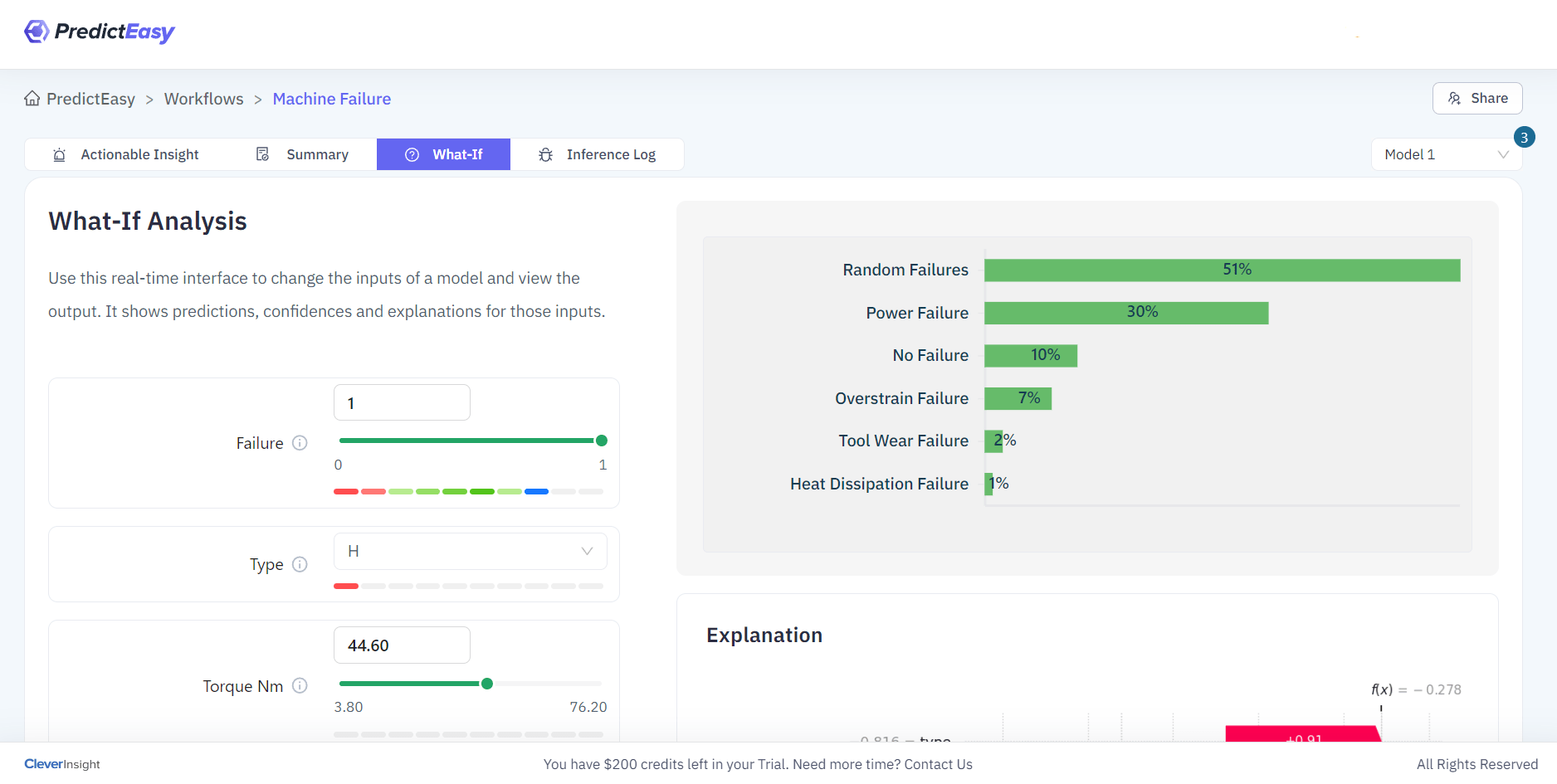
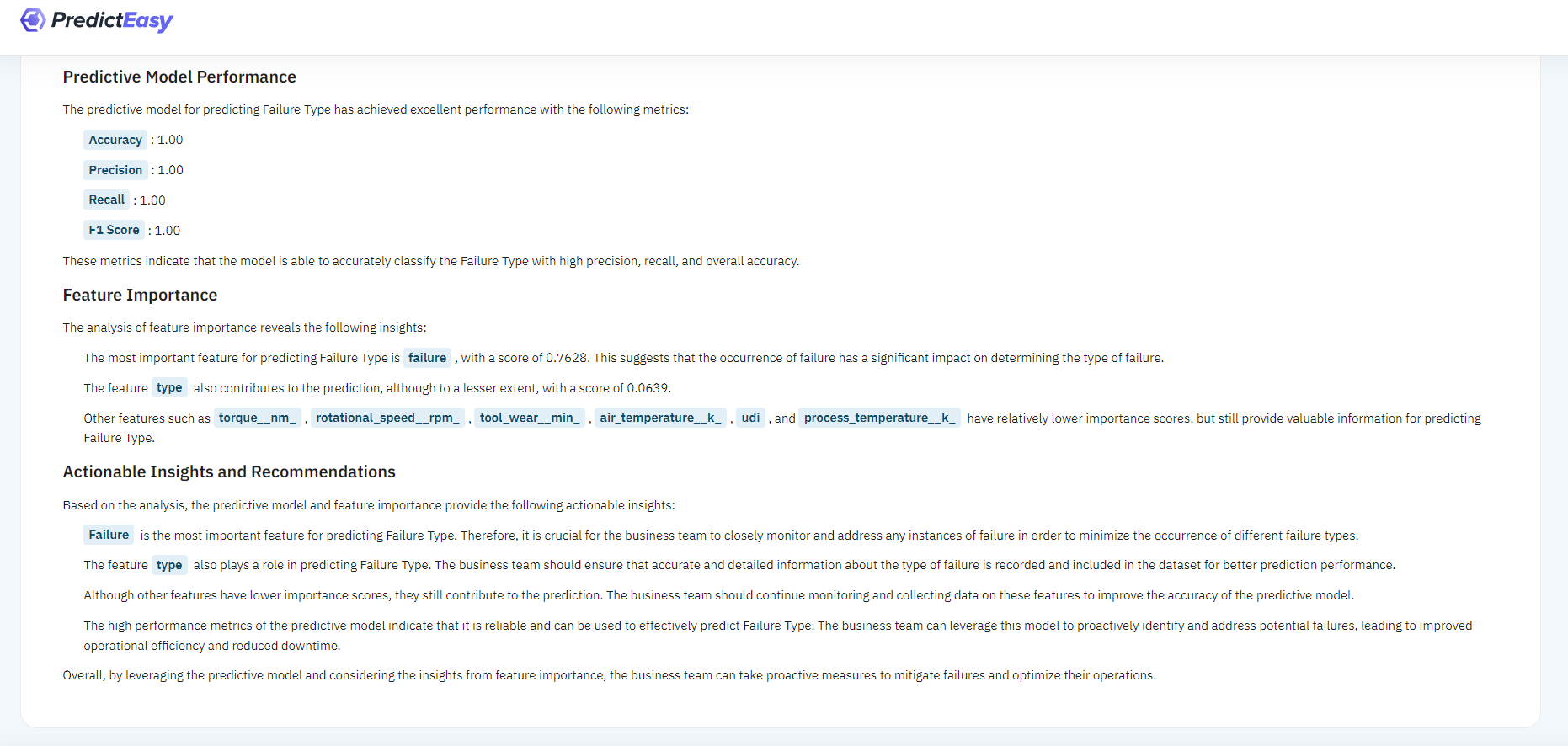