Leveraging advanced machine learning algorithms, credit card transactions are monitored in real-time to detect and prevent fraudulent activities, ensuring enhanced security for cardholders.
The Challenges
Detecting evolving fraud techniques is a constant challenge for credit card fraud detection systems. Imbalanced data, where fraudulent cases are outnumbered, poses a hurdle for machine learning models. Real-time processing is crucial, making it challenging to implement machine learning models without causing delays. Adapting to new fraud patterns requires continuous training and updates for machine learning models.Balancing machine learning for fraud detection with customer privacy and data protection regulations presents significant challenges.
What did PredictEasy do...
PredictEasy uses statistical tests like the Pearson Correlation Coefficient and machine learning model building to improve decision-making and prediction capabilities across different fields. The platform analyzes relationships, identifies patterns, and understands variations through tests such as Spearman Rank Correlation, Chi-Square Test, and ANOVA. These tests reveal connections between features, uncovering correlations and significant differences.
In machine learning model building, PredictEasy utilizes historical data to predict future outcomes. By training algorithms on relevant datasets, the models learn patterns and correlations, providing user-friendly predictive analytics without extensive coding. The combination of statistical tests and machine learning enhances predictive capabilities, allowing users to make informed decisions, plan for scenarios, and gain actionable insights.
PredictEasy includes a real-time What-if Simulator, enabling dynamic input adjustments and observation of corresponding probability values. This simulator aids in exploring scenarios, understanding potential changes, and guiding decision-making in dynamic situations.
In summary, PredictEasy offers a comprehensive and user-friendly platform for data analysis, prediction, and decision-making by combining statistical tests and machine learning model building.
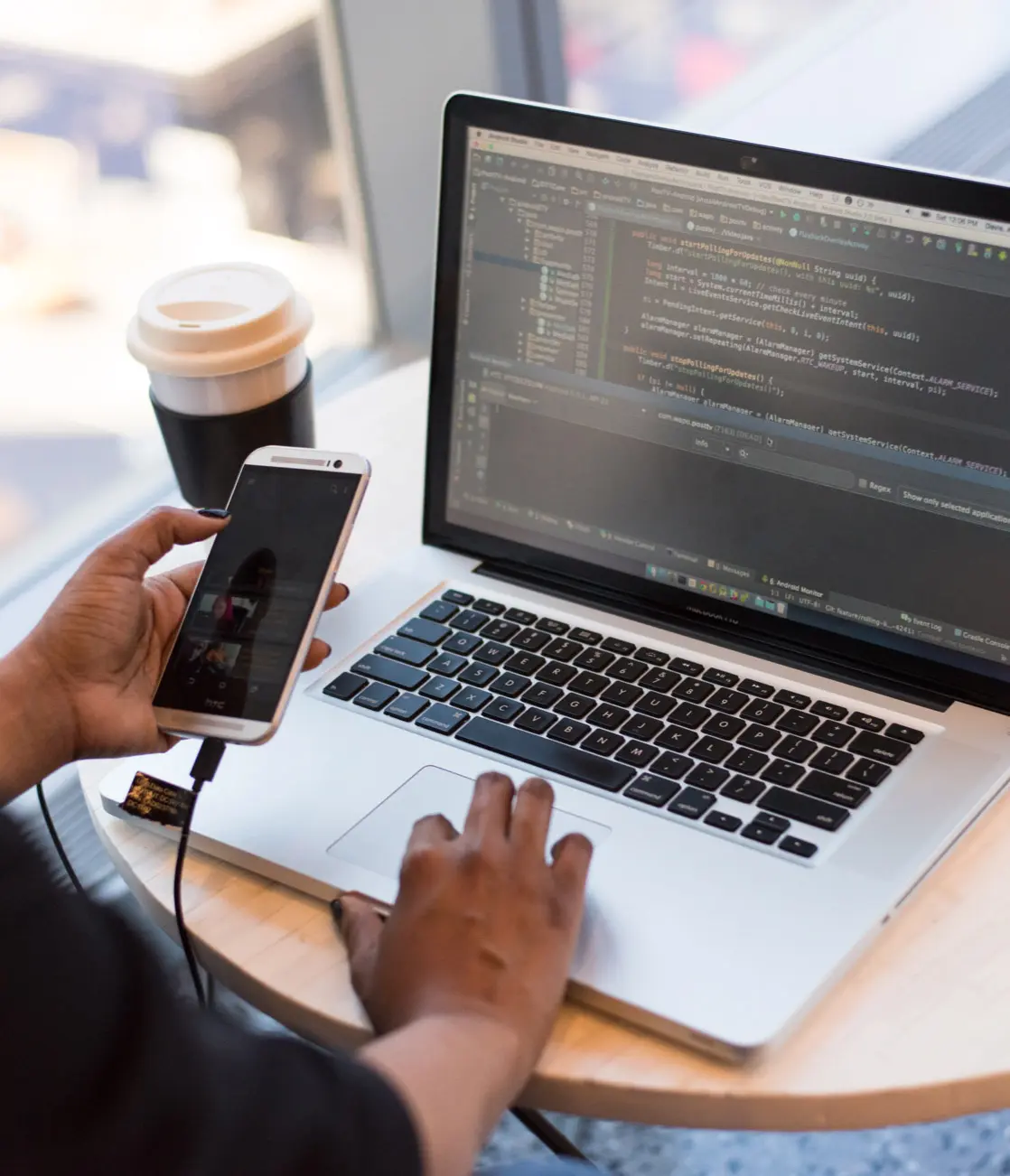
The Results
The combination of statistical tests and machine learning enhances PredictEasy's predictive capabilities, enabling users to make informed decisions, plan for scenarios, and gain actionable insights.
The combination of statistical tests and machine learning enhances PredictEasy's predictive capabilities, enabling users to make informed decisions, plan for scenarios, and gain actionable insights.
With features like the real-time What-if Simulator, PredictEasy allows dynamic input adjustments, aiding in scenario exploration, understanding potential changes, and guiding decision-making in dynamic situations.
With features like the real-time What-if Simulator, PredictEasy allows dynamic input adjustments, aiding in scenario exploration, understanding potential changes, and guiding decision-making in dynamic situations.