Telecom churn refers to customers switching from one telecom service provider to another, often due to dissatisfaction or better offers. Machine Learning plays a crucial role in predicting and mitigating churn by analyzing patterns and identifying factors that contribute to customer attrition, enabling companies to proactively address issues and enhance customer retention strategies.
The Challenge
Diverse Customer expectations in network quality, customer support, and pricing pose challenges for consistent customer satisfaction. Intense competition with rival telecom companies offering attractive promotions to retain subscribers.
Staying technologically relevant and ensuring regulatory compliance adds complexity, requiring ongoing adaptation. Acquiring and integrating high-quality data is crucial for accurate churn predictions.
Balancing the complexity of ML models is challenging, as intricate models may be harder to interpret. Real-time analysis using the What-If to know possibility of Churn and efficient data processing.
Maintaining a delicate balance to uphold customer trust is essential amidst the challenges on both the telecom and ML fronts.
What did PredictEasy do..
What-if analyses highlight user concerns and subscription impact on churn probability.
Explainable AI-driven analysis identifies crucial churn factors for targeted efforts.
Actionable insights recommend issue resolution and improved streaming experiences. PredictEasy’s AI model helps pinpoint high-risk users based on billing preferences and tenure.
Strategic recommendations include improving technical support and incentivizing yearly subscriptions.
Leveraging predictive accuracy to address potential churn is advised. Supported by robust statistical tests and PredictEasy’s AI insights.
Recommendations offer a comprehensive understanding of customer behavior and empowers businesses to make informed decisions and enhance overall customer experience.
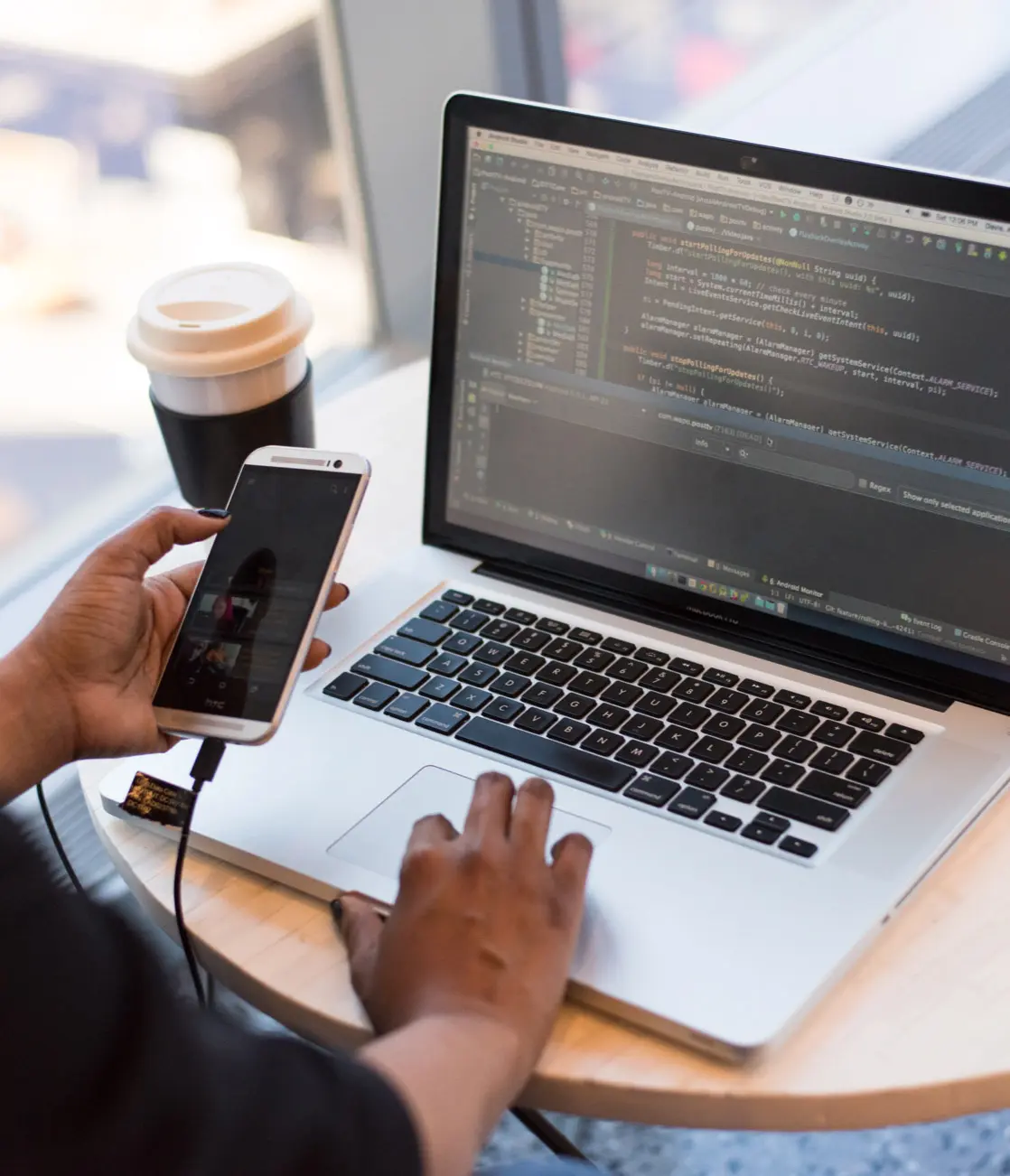
The Results
Unresolved Issues Impact Churn:
Effective issue resolution is crucial for customer retention, as users with unresolved concerns are more likely to switch to another service provider.
Billing Method and Churn:
The choice of billing method matters, as customers opting for electronic bill payments exhibit a higher churn rate compared to those using automatic credit card or bank transfer payments.
Multiple Connections and Complaints:
Overall satisfaction across various connections plays a role in churn decisions, as customers with multiple connections are less likely to switch unless faced with numerous complaints.
Streaming Plan Subscribers:
Users subscribed to streaming plans show a slightly higher likelihood of churn, potentially indicating dissatisfaction with the quality of the streaming service.
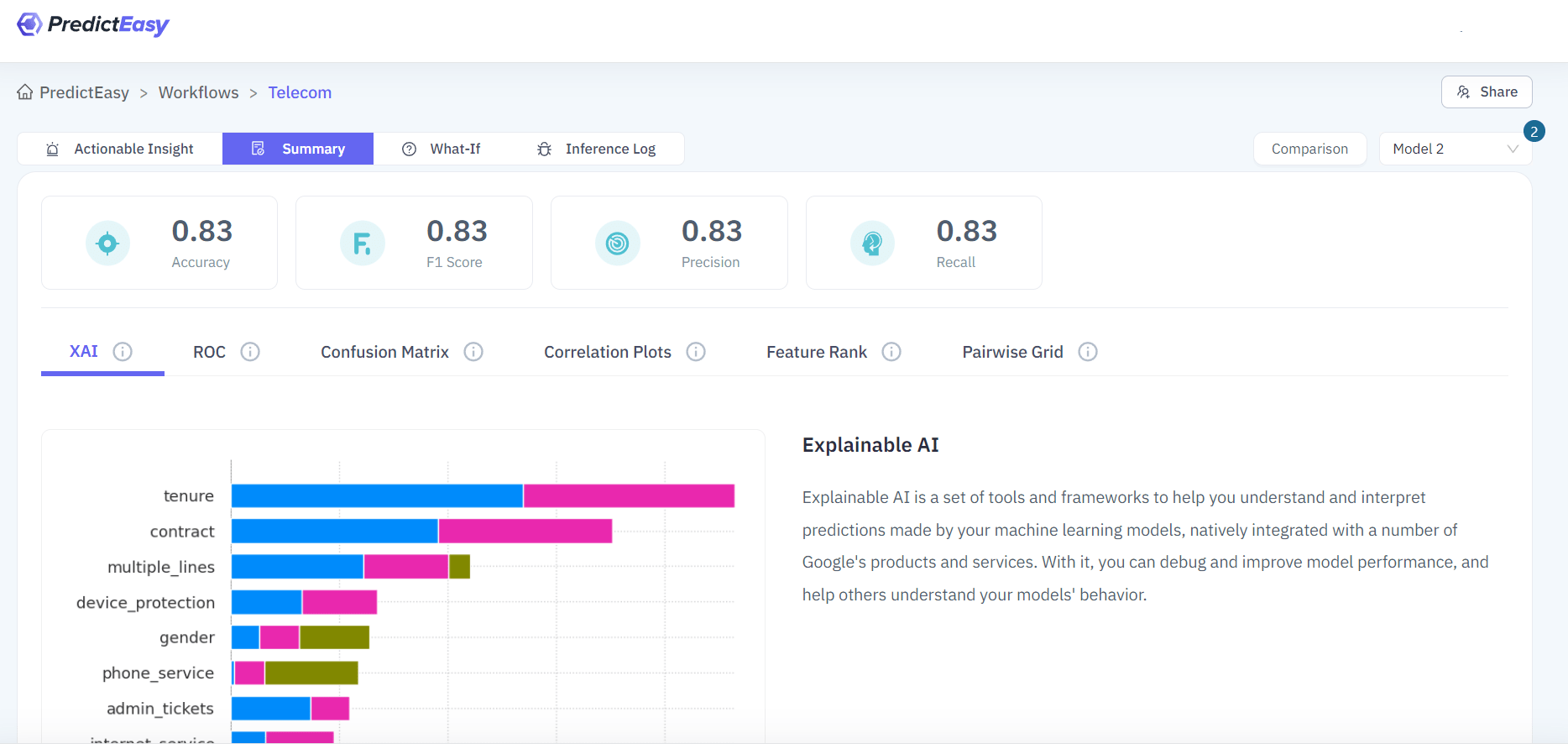
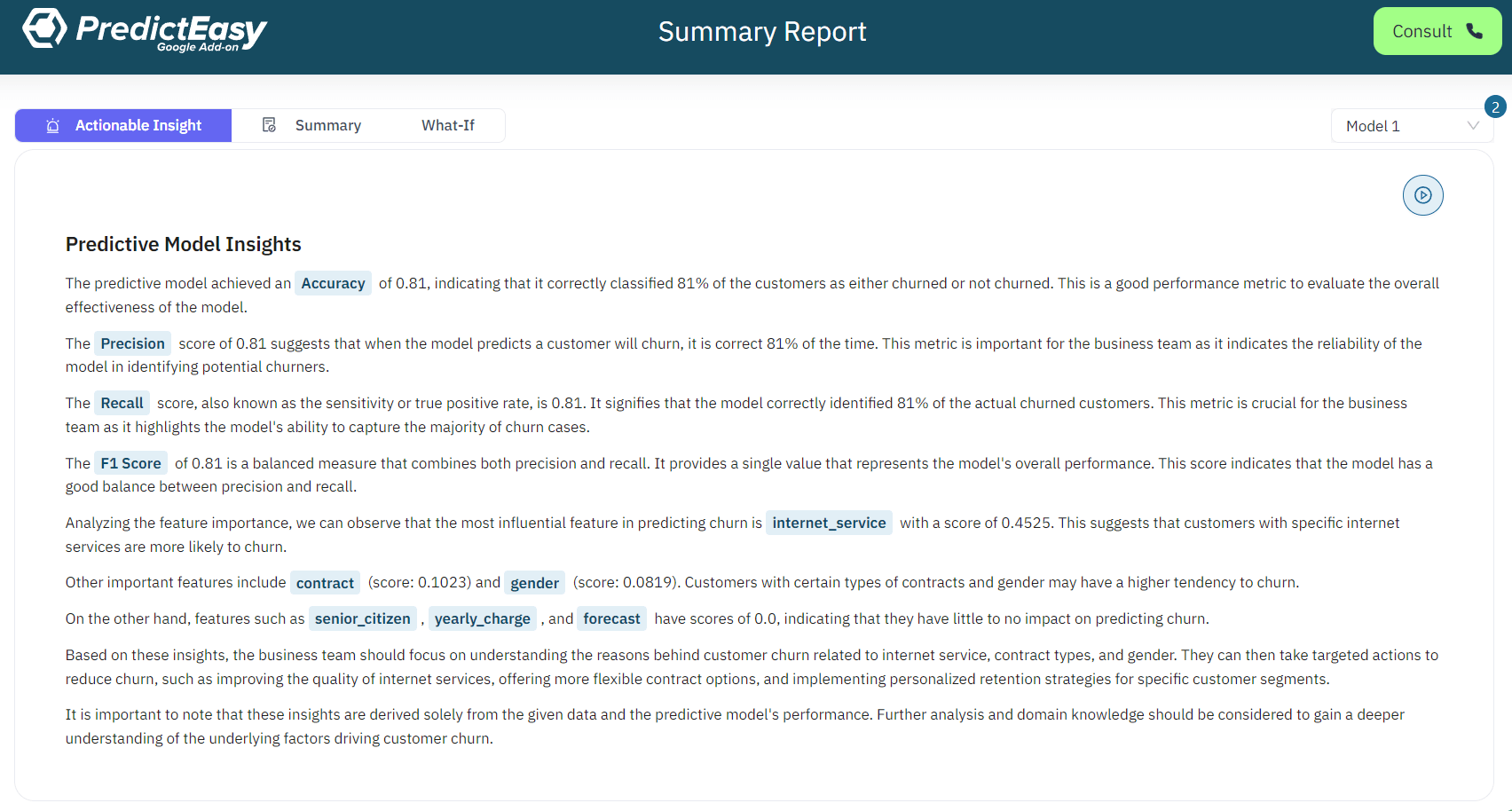